Things we do to - sometimes unknowingly - slow down our Data
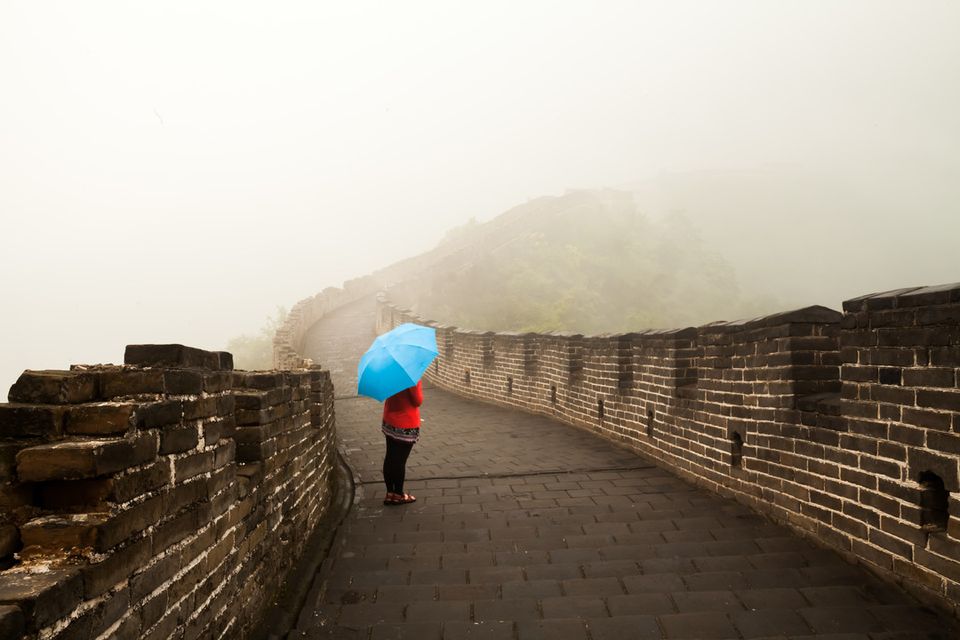
It is yet another one of those days in the office: my director had just endured a severe dressing down from the CEO and as the usual cascading ritual was moving around the hierarchy like a ricochet, I had the unfortunate situation of our coffee rounds coinciding.
After getting a generous helping of bitter medicine, my director goes on to add, "Do you know the honest problem with Data departments, like ours?", a question in no way positioned like it expected a verbal response. "The trouble is, we get data flying in at such speeds - from a variety of sources - and we know this speed is increasing all the time; with the increase in the use of digital channels and with the increasingly demanding customer, who wants it all now! And what do we do? We slow it all down - with our myriad of tediously tortuous processes, with our spaghetti ETL, and with our complex byzantine data warehouses!".
While on the surface this might have come across somewhat castigating, the underlying message was clear: as while by no means the intention at the start of its journey, our data landscapes have indeed become riddled with pace annihilating steps. Some of these steps had effectively become hurdles - or at times even brick-wall-like barriers - to both data flows and data products engineering.
The period of Radical Candor that followed was, however, to deliver a number of insightful revelations - about our landscape - which we had, at the time, thought absolutely normal and taken for granted.
Some of such discoveries - about our data landscapes - included:
- Our endless race towards database integrity - Enforced data relationships (such as via foreign keys) meant that data was often stuck partway through the pipeline (for instance, if a service was sold before its master data had been propagated through the data warehouse, then all subsequent sales transactions would be stuck or if a customer moves to an address yet to be updated on the post office's databases, then their customer data would struggle to be pushed into tables and data sets).
- The need for dependency management - If customer complaints data arrived while the customers details are being blocked (e.g., because their new address details were not on our databases), then this data would be stuck in a holding pattern and would not provide the kind of early visibility opportunity desired. Indexes, which helped improve search performance, were also a nightmare to maintain.
- Our winless quest for data quality - It was clear that such issues were impossible to completely eradicate, however, lots of processes put in place to arrest this either slowed the pipeline down or potentially caused complete data blockages.
- The stone cottage rigidity of our data structures - This meant that any required change to structure, not only presented significant intrinsic "data modelling" challenges, but posed broader challenges across the entire data pipeline.
- Tight coupling - This change management nightmare meant that even where not driven by demand for new data or analytics products (or features), changes to source systems were potentially significantly disruptive.
"So, we are clearly in a bit of a mess. The business is forecasting astronomical growth whilst our landscape struggles to cope with today's demands!", a colleague declares, stating the petrifyingly obvious. After a number of fairly impassioned debates - leading to nowhere in particular (at least not in a hurry), a most unlikely voice demands, "So, rather than sit here agonising about our collective pain-inflicting hindsight, should we not channel our valuable brain power towards getting ourselves out of this mess?".
Below are three of the most tangible - eventual - strategies for radically moving our data landscape in the right direction:
- A move to(wards) the Cloud - it was quite clear that the Cloud provided the sort of opportunity to provision and to scale that was, so desperately needed but, absolutely unattainable within our self-managed data centre infrastructure.
- Enabling a seamless Cloud transformation via a transition friendly hybrid architecture - this was essential as a big bang transformation was not only inconceivable and unviable but would not deliver the kind of tangible benefits desperately needed, and within an acceptable timeframe. This was only achievable by iteratively delivering value - in manageable chunks.
- Reimagining the Big Data warehouse - a previous piece touched on some simple tips for speed and agility. It was clear that simply lifting and shifting, our current data pipelines or target data structures, could only offer limited short term advantages - at best. We needed to rethink "how" do we build sustainably resilient data platforms and data services?
Credits
More on Radical Candor from @Kim Malone Scott https://www.linkedin.com/in/kimm4/
Member discussion